While Artificial intelligence (AI) models that can play games have existed for decades, they usually focus on just one game and always want to win. With their most recent creation, a model that learnt to play various 3D games like a human while also trying its best to comprehend and follow your vocal directions. Google DeepMind researchers had a different goal in mind.
Of course, “AI” or computer characters are capable of this sort of thing. But they’re more akin to game features—that is, non-player characters (NPCs) that you can manage indirectly through official in-game commands.
DeepMind trained its SIMA (scalable instructable multiworld agent). On countless hours of human gaming footage, without access to the actual game code or rules. Data labelers provided annotations. And the team used this data to train the model to recognize specific visual representations of objects, interactions, and activities. They also captured videos of players giving each other game instructions.
For instance, it might recognize an action as “moving forward” by observing the movement of pixels on the screen. Or, it might identify the action of “opening” a “door” when a character approaches something that looks like a door and uses something that resembles a doorknob. These minor actions or events, taking just a few seconds. Involve more than merely choosing an item or pressing a key. Such little things, actions, or occasions that take a few seconds but are more than simply selecting an item or hitting a key.
Researchers trained an AI on games like Goat Simulator 3 and Valheim, aiming to generalize gaming skills. They found that AIs trained on multiple games performed better on new ones. Despite challenges from unique game mechanics, indicating that extensive training could overcome most learning barriers.
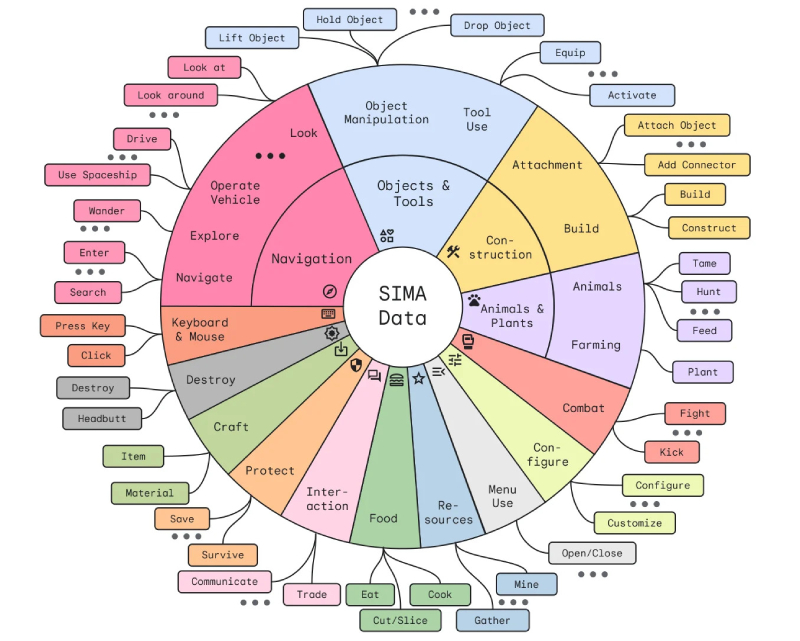
Advancing Towards Dynamic AI Gaming Companions
In addition to making significant progress in agent-based AI. The researchers hope to develop a more organic gaming companion than the rigid, hard-coded ones we currently use.
Tim Harley, one of the project’s leads, said, “Instead of having a superhuman agent you play against, you can have cooperative SIMA players beside you that you can give instructions to.”
They have to learn skills much as humans do because, when they play, all they can see are the pixels on the game screen. However, this also implies that they are able to adapt and exhibit emergent behaviors.
You might be wondering how this compares to the simulator approach, a popular technique for creating agent-type AIs. In this method, a largely unsupervised model experiments wildly in a 3D simulated world that runs far faster than real time. Enabling it to learn the rules intuitively and create behaviors around them with a significantly reduced amount of annotation work.
The “reward” signal for the agent to learn from in traditional simulator-based agent training. Is the game or environment’s win/loss in Go or Starcraft, or the “score” in Atari games. According to Harley, who told TechCrunch, this method was used for those games and produced amazing results.
“We do not have access to such a reward signal in the games that we use. such as the commercial games from our partners,” he went on. Additionally, we are looking for agents that can perform a broad range of activities defined in open-ended language. It is not practical for every game to assess a “reward” signal for every possible objective. Rather, we use imitation learning to teach agents with text-based goals by observing human behavior.
An inflexible reward system limits an AI’s choices to maximizing scores. Training it to value abstract concepts fosters creativity. Other companies explore this unrestricted cooperation; for instance, using chatbots for NPC discussions. AI also simulates unplanned interactions, advancing agent investigation.